
“Artificial General Intelligence” (AGI) is, to put it mildly, a controversial concept in AI research. The word is of recent vintage, dating to about the turn of the 21st century, and comes from an attempt to make clearer an idea that has circulated in AI since, arguably, before the invention of computers. It’s gone by a lot of names over the years: “true AI,” “real AI”, “strong AI,” “human-equivalent AI,” etc. The history of attempts to define it in some testable way is, to say the least, not encouraging, but it doesn’t seem to stop anyone from trying.
The Curses of AI
Artificial intelligence is a cursed field.
The word was coined by John McCarthy in 1956, in large part to distinguish it from “cybernetics,” a label associated with Norbert Wiener, who saw it as an interdisciplinary field with political implications that did not appeal to McCarthy and his colleagues. So, from the very beginning, AI has been associated with intellectual hubris and disinterest in social consequences, willfully distancing itself from the humanities — the study of human works — while purporting to build machines that do the work of humans.
That is one of AI's great curses: There is an expansive, argumentative, routinely acrimonious literature on the nature of intelligence and human intellectual abilities, dating back centuries and even millennia, and discussions of artificial intelligence reference it approximately never. How exactly are you going to discuss “artificial intelligence” if you can’t say what it means for something to be intelligent, or how you would demonstrate that?
Agüera y Arcas of Google and Norvig are not especially clear on how they’ve defined “general intelligence” despite having a section titled “What Is General Intelligence?”
Broadly, their claim seems to be:
- General intelligences can do more than one kind of thing.
- General intelligences can do things that their designers didn’t specifically intend for them to be able to do.
- General intelligences can do things that are specified in your instructions to them rather than their programming.
This is my summary, and perhaps I have misunderstood them, but the section where they explain this goes on a number of tangents that make it hard to identify what they intend to say. They appear to be using the label “general intelligence” to contrast with “narrow intelligence,” another new term (I can’t find reference to it before the 2010s) that seems to have the same relationship to what we used to call “weak AI” as “general intelligence” has to “strong AI.”
They define it as follows:
Narrowly intelligent systems typically perform a single or predetermined set of tasks, for which they are explicitly trained. Even multitask learning yields only narrow intelligence because the models still operate within the confines of tasks envisioned by the engineers.
They contrast this with “general intelligence”:
By contrast, frontier language models can perform competently at pretty much any information task that can be done by humans, can be posed and answered using natural language, and has quantifiable performance.
This approach poses some obvious problems. It defines artificial general intelligence to exist only when engineers have poor imaginations. That doesn’t seem quite right.
The most frequently cited example of an AI model performing outside of its explicit training (a.k.a. zero-shot learning) is the “zebra” example: Let us say we have a multimodal AI model that has never had a picture of a zebra or mention of a zebra anywhere in its training data. So we tell it that a zebra is a horse with stripes, and then we present it with pictures, some of zebras, some not, and ask it if each one is a zebra.
The current generation of multimodal models is capable of doing a good job of this.
I don’t know if this is exactly what Agüera y Arcas and Norvig have in mind as performing outside of “the confines of tasks envisioned by the engineers” because they give no examples, nor does the article they link to on this subject. (”A general AI model can perform tasks the designers never envisioned.”) But this kind of zero-shot learning does seem to be what they mean.
However, it's not clear that this really is an example of going outside of the tasks envisioned by the model's designers. Given that the model was explicitly trained to recognize horses and stripes and to connect the features it finds in images to natural language words and statements, it was explicitly trained to handle all parts of the problem. So is it right to say it’s exceeded the bounds of “narrow intelligence?”
Other zero-shot learning examples suffer from the same problem.
Also, unexpected correct results from computers have been around for some time. As an example, this article from 1999 shows a logistic planner in a NASA space probe doing something that not only was correct and unexpected but that the engineers could never have had in mind when they designed it.
But there’s an even larger problem with defining artificial general intelligence this way.
Let’s consider the large language models, like ChatGPT. What were the tasks its engineers designed it to do? Only one task: Add the next word to a text.
The web interface to ChatGPT obscures what happens behind the scenes, but ChatGPT works like this:
Iteration 1:
User Input: Knock, knock.
GPT Output: Who’s
Iteration 2:
GPT Input: Knock, knock. Who’s
GPT Output: there
Iteration 3:
GPT Input: Knock, knock. Who’s there
GPT Output: ?
Return to user: “Who’s there?”
That’s all it does! You see it writing poetry, answering questions, or whatever else you like, but under the surface, it’s a sequence of identical simple operations to append a word to a text.
We could say that ChatGPT does just one task, the one it was explicitly designed for.
So, is this a single-task ability or multi-task abilities? Is it doing more than the engineers designed it to do, or only exactly the one thing they designed it to do?
It depends on how you choose to look at it. This kind of ambiguity is not trivial to resolve.
The article highlights “[a] healthy skepticism about metrics for AGI” as a legitimate concern. However, the problem with “general intelligence” isn’t metrics and thresholds. It’s definitions. AI’s lack of clear definitions of its terms is the direct cause of AI’s second great curse: Moving goalposts.
Goalpost-moving is so central to AI as an enterprise that Professor Koller introduced me to it in her introductory class long ago, I think on, like, Week 3.
Every time researchers solve some problem in AI, it’s redefined as not needing intelligence. As Pamela McCorduck puts it in her influential history of AI:
Practical AI successes, computational programs that actually achieved intelligent behavior, were soon assimilated into whatever application domain they were found to be useful, and became silent partners alongside other problem-solving approaches, which left AI researchers to deal only with the “failures,” the tough nuts that couldn’t yet be cracked. Once in use, successful AI systems were simply considered valuable automatic helpers. [McCorduck 2004]
Or, in shorter words, attributed to Larry Tesler (the inventor of copy-paste functionality):
AI is whatever hasn't been done yet.
AI is a Red Queen’s Race where no matter how fast you run, the finish line moves away even faster. The history of AI is a history of failure because we excise the successes from it. Never mind that AI research was already years ago bringing us decent spellcheckers, functional machine translations, and visual object recognition, among other accomplishments of modern technology.
Or that half the world today runs on logistic planners that started life in the 1960s as AI research in automated theorem proving. Yes, your “supply chain” is already AI from end to end. AI didn’t get rid of the drudgery of trucking and manually loading and unloading goods. It got rid of the comfy office job of scheduling.
In the history of AI goalpost-moving, Agüera y Arcas and Norvig’s contribution is somewhat novel: We’re used to moving the goalposts of “real” AI to make them ever harder to reach. They’ve moved them to say we’ve already passed them.
The cause of the moving goalposts is more complex: AI is cursed with bad incentives. It lends itself to hype. We might blame this on the grants process and academic pressures to overpromise, or on late capitalism and the way the tech investor class likes to chase after the latest shiny thing in hopes of owning a “disruptive” new technology.
But I put a lot of the blame on AI’s final curse: Science fiction.
Now, understand me: This is not a criticism of the makers of science fiction; it’s a criticism of its consumers. I have been watching and reading science fiction since I was five years old, and I love it. It’s a popular genre, beloved by millions, if not billions, of people.
But the ideas people have in their heads about AI, including the people who do AI research, have been so thoroughly colonized by fictitious machines that it has become difficult to separate legitimate debates from the dramatic visions of novelists and movie makers.
Ideas about “artificial general intelligence” or “real” AI owe far more to science fiction than to science. It’s not the responsibility of the Issac Asimovs, the Stanley Kubriks, or the James Camerons of the world to police their imaginations or ours. It is the responsibility of AI professionals — researchers, marketers, investors, and corporate frontmen — to understand and make clear that “positronic brains,” HAL 9000, and killer robots with inexplicable Austrian accents have no place in engineering.
We move the goalposts in AI because every accomplishment ends up disappointing us when it doesn’t live up to our vision of AI. Rational beings might interrogate their visions of AI rather than move around the goalposts and say: “This time, it’s different!”
Humans have deep prejudices that they struggle to recognize. Agüera y Arcas and Norvig recognize this in their mention of the “Chauncey Gardiner effect.” For younger readers who don’t know the works of Peter Sellers and Hal Ashby, we might also call this the “Rita Leeds effect” after Charlize Theron’s role in season three of Arrested Development. It’s the same schtick.
Until the 1970s, there were serious social scientists who believed that black people, at least American ones, were systematically intellectually stunted and pointed to the way they spoke English as proof. Even today, plenty of people view African American Vernacular English (usually abbreviated as AAVE because who has time to spell that out?) as “broken” or “defective” or indicative of poor education.
Among linguists, no one of any importance today thinks that. We recognize AAVE as a fully functional variant form of English, just as well-suited to accurate and effective communication as standard English or any of its other variants. It has no relationship to cognitive abilities whatsoever.
Yet, the prejudice against it remains.
When we evaluate ChatGPT as “real” AI, or at least on the path to “real” AI, we are demonstrating the same prejudice, turned around. That should give us some pause. It should lead us to ask if anything we think makes software intelligent is really intelligence or just manipulating our prejudices. Is there any way to objectively judge intelligence at all? We may never be able to fully dismiss our prejudices.
It does not take a lot of interaction with ChatGPT to see it fall short of our science-fiction-based visions for AI. It can do some neat tricks, maybe even some useful ones, but it’s not WALL-E, or C-3PO, or Commander Data. It’s not even Arnold Schwarzenegger's iconic but not terribly verbal T-800, although I don’t doubt you could readily integrate ChatGPT with a text-to-speech model that has a thick Austrian accent and pretend.
Changing the labels on things doesn’t change things, at least not in any simple way. We could move the goalposts for “artificial general intelligence” in such a way that we could say we’ve already accomplished that, but that will not make today’s AI models more satisfactory to people looking for HAL 9000. It would just render the label “general intelligence” meaningless.
People would still want something we can’t give them. Something we might never be able to give them.
Are Humans Still Special?
Among the strawmen Agüera y Arcas and Norvig take on in a whole section is “Human (Or Biological) Exceptionalism.” People believe all kinds of things, but unless you count religious notions of the “soul”, it is hard to believe this is a commonly held position among AI professionals. More likely, most of them don’t think about it at all.
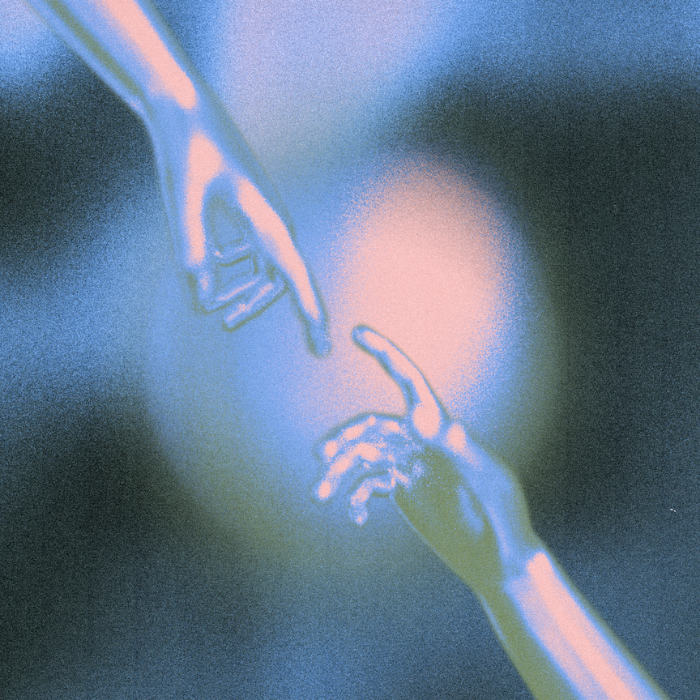
The lesson we should be learning from recent AI is that it is difficult to identify a human skill that is absolutely impossible for a machine to perform. We cannot make AI models do everything a human can do yet, but we can no longer rule anything out.
This realization should end some of the bad ideas we have inherited from the past. We know today that animals have languages much like our own and that computers can learn language with a shocking facility. Noam Chomsky may not be dead yet, but ChatGPT has put the final nails in the coffin of his life’s work.
We have known since Galileo that humanity does not live in the center of the universe. We have known for roughly two centuries that the universe existed for billions of years before people. We have known for some time that humans are not made special by the use of reason and language, as Descartes thought, as we find both among animals and note their frequent absence in humans.
AI research is removing the idea that humans are special because of some trick we can do, but that does not remove the specialness of humanity. Consider that we celebrate people who can run a mile in under four minutes. Yet, my 20-year-old cheap French-made car can do a mile in about 30 seconds if I really put my foot on the pedal.
We care about human accomplishment in ways that differ from how we care about pure performance benchmarks. We’ll still prefer our human performers over AI, for the same reason I don’t win Olympic medals with my old Citroën.
We imagine AI taking away the drudgery of human life, but we do not yet have robots that can restock shelves at Walmart. On the other hand, we now have AI models that can sing like Edith Piaf and, if someone really tried, could probably dance like Shakira or play the guitar like Jimi Hendrix.
This is progress. It’s an accomplishment of significant value. But it’s not the AI we wanted. Unless we rethink the whole program of AI research, we’ll need to move the goalposts again.
Real AI instead of “Real” AI
“Past performance is not a guarantee of future returns.” Maybe it’ll be different this time. Maybe we won’t dismiss our successes as not “real” intelligence. Maybe we’ll get over our science fiction dreams for once. Maybe this hype cycle won’t lead to another AI Winter.
Maybe, but I won’t bet on it.
Someday, we might have something that looks more like our visions of “real” AI. Perhaps in 50 years? 100? History does not encourage optimism about it. But regardless, it won’t happen in a timeframe that pays any bills.
Moving around the goalposts of AI won’t change that.
Our interest is business cases. We want AI to add to your bottom line. At CMON AI, we want to hear about your business processes and discuss how really existing AI models can fit into them. We know the limits of this technology intimately, but we also know its genuine potential.
This article was sourced from jina.ai